Clinical Informatics Approach
Elimu approaches challenges from both a clinical perspective and an informatics perspective. The Disease Management Lifecycle guides our clinical perspective while the Data to Knowledge Foundation drives our informatics perspective.
Clinical
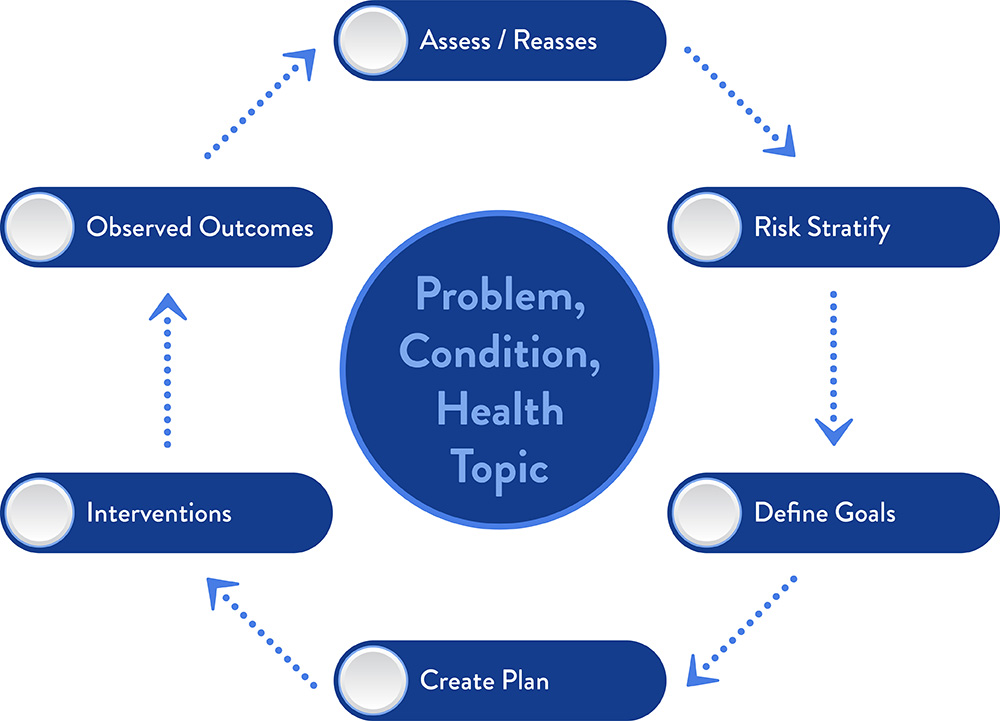
Disease Management Lifecycle
The Disease Management Lifecycle guides Elimu’s clinical approach, enabling us to apply knowledge at the correct point of care.
Value sets, data normalization, machine learning, and FHIR are all great tools, but where and how do they fit into a clinical workflow to drive better outcomes and meet other clinical objectives? The Disease Management Lifecycle provides a framework for leveraging knowledge and invoking the “five rights” (right information, to the right person, in the right format, through the right channel, at the right time in workflow).
Consider cancer screening as an example, where a clinical objective is to improve the cancer screening rate. Risk factors might include family history, smoking history, age, and genetic variants. Knowledge components define a computable method for identifying patients at risk; for differentiating those that have and have not been screened; for calculating quality indicators; etc. These components might be deployed via a large-scale patient outreach program, a point of care decision support, or via another customer-specific workflow. Elimu assures that "all the dots connect":
- The risk factor definitions align with data captured at the patient level
- The reporting systems can "see" relevant information captured in the patient health record
- The decision support rules and order sets support the goals/objectives of the care pathways for disease management and health maintenance
- We do all this by using leveraging our terminology services, decision support services, reference content mapping/tagging and enhancement, and knowledge inference services
Informatics
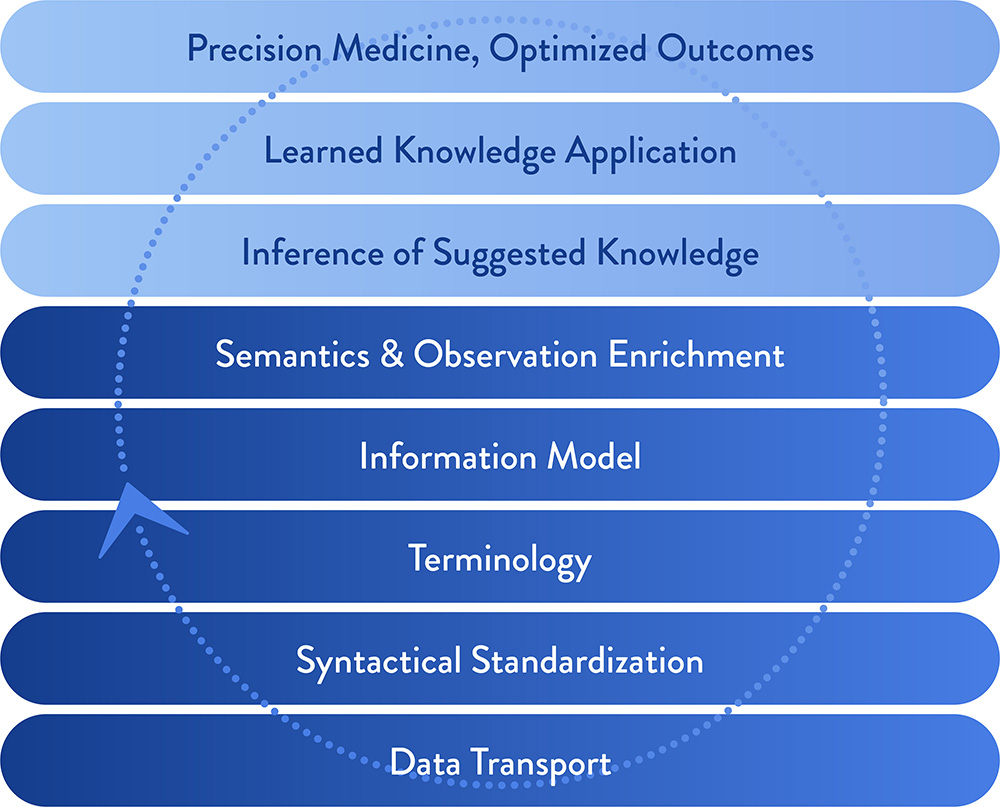
Data to Knowledge Foundation
The Data to Knowledge Foundation drives Elimu’s informatics approach, enabling us to turn data into knowledge.
At the bottom of the Foundation sits data. As that data is normalized against defined terminology and information model specifications, it becomes amenable to enrichment. Inferences can then be drawn from the enriched data, and those inferences can be applied in personalized ways, to optimize care. This is an iterative (“learning”) process, where data is continuously driving the data to knowledge process.
Consider pharmacogenomics as an example. Data (e.g. patient genotype and phenotype) are obtained, communicated per specifications (e.g. HL7 FHIR, RxNorm, SNOMED). Inferences (e.g. genome-wide association studies) can suggest drug-gene associations. These associations can be deployed through an application (e.g. pharmacogenomic clinical decision support) as a way of personalizing care.
While many contribute components to the Data to Knowledge Foundation, Elimu will ensure that all the building blocks are cohesive, and that the gleaned knowledge can be deployed towards clinical objectives.